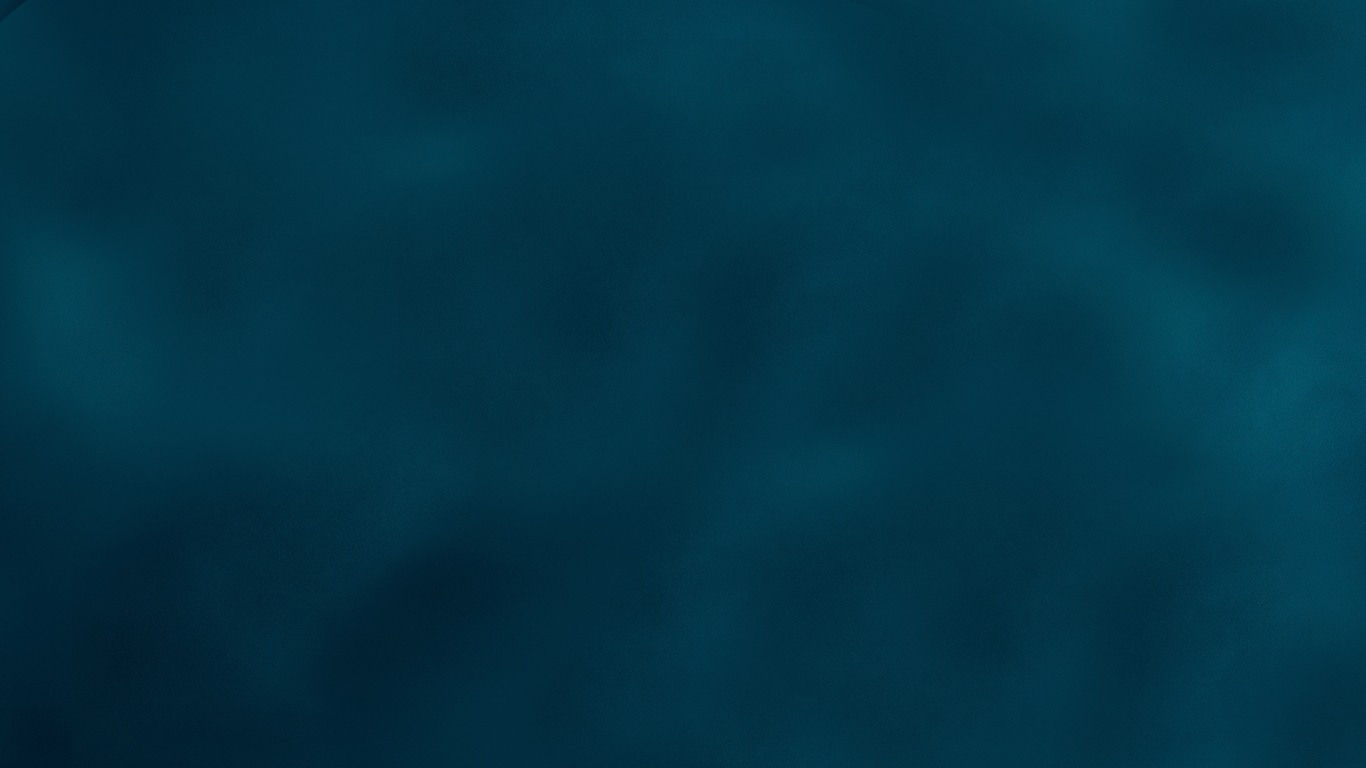

When people consider artificial intelligence they often think of examples of super computers like IBM’s Watson or Deep Blue. These types of AI are not actually capable of learning themselves, but instead reference previously learned information in large databases and use neural networks to build an appropriate response. Neural networks are how the information in the database is presented to the AI, and are modeled after how humans learn. Although Watson and Deep Blue are good examples of the power and speed of recent advances in AI, they may be considered a bit primitive in their approach to learning.
Most modern AI systems use machine learning and multiple passes or generations to learn how to complete a task. In the AI dinosaur example, the AI was incentivized to reach the farthest possible distance in the game without explicitly being programmed how to do so. In early generations the AI realizes that constantly jumping is not a good strategy and learns only to jump when an obstacle is present. With every new generation the AI learns from past failure and adjusts its strategy to complete the designated task. As the simulation continues the AI becomes more and more intelligent and eventually becomes an expert at the task. This trial-and-error approach is widely used in the development of modern AI technology.
